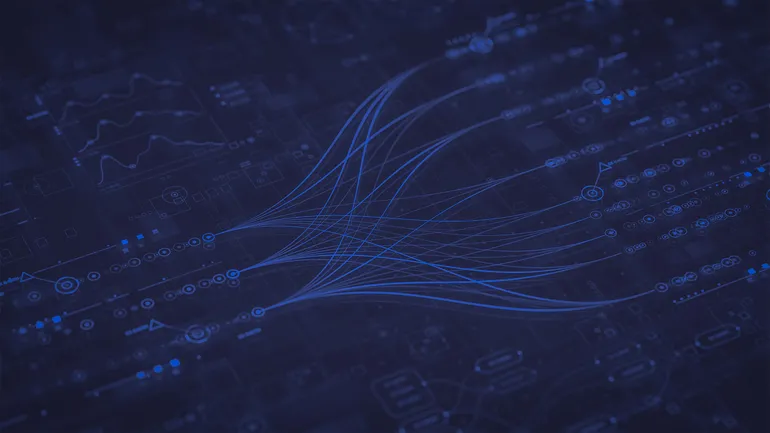
Welcome to the first installment of our three-part series exploring the groundbreaking impact of AI on DICOM data migration. Following Enlitic’s acquisition of Laitek in October 2024, a new era of medical imaging data management has begun. This series will unpack how the fusion of Laitek’s data migration prowess with Enlitic’s AI innovations is reshaping healthcare data workflows, providing faster, more accurate, and less disruptive solutions to long-standing challenges.
The world of medical imaging is undergoing a profound transformation. The engine driving this change? Artificial intelligence. While AI’s diagnostic capabilities often steal the spotlight, a quieter revolution is occurring behind the scenes: the way healthcare data is managed, migrated, and ultimately, made useful.
For years, hospitals and clinics have wrestled with the challenge of moving vast archives of medical images from outdated systems to newer, more efficient platforms. This process, known as DICOM (Digital Imaging and Communications in Medicine) data migration, is far from simple. It’s a complex, time-consuming, and often risky undertaking that can disrupt clinical workflows and strain IT resources.
Imagine a hospital deciding to upgrade its Picture Archiving and Communication System (PACS). This system, the heart of medical imaging, stores millions of X-rays, CT scans, MRIs, and other diagnostic images. Migrating this data to a new PACS is like moving an entire library, one book at a time, while ensuring each volume is correctly cataloged and shelved in the new location. The stakes are high: any errors or omissions could have serious consequences for patient care.
Traditionally, DICOM data migration has been a manual and labor-intensive process. IT teams would painstakingly extract data from the old system, cleanse it of errors and inconsistencies, and then load it into the new system. This process could take months, even years, to complete, and was often plagued by inaccuracies and data loss.
But now, AI is stepping in to streamline and improve every stage of the migration process.
Uncovering Hidden Insights
The first step in any data migration project is to understand what you have. This involves creating a detailed inventory of all the images, studies, and patient information stored in the legacy system. In the past, this was a tedious and error-prone task.AI is changing that. By using sophisticated algorithms to analyze the DICOM data, AI can quickly and accurately identify the number of studies, types of studies, and the total number of images for each patient. It can also detect inconsistencies and errors in the data, such as missing information or incorrect labels.
More importantly, AI can validate the consistency between the PACS database and the hospital’s Electronic Medical Record (EMR). The EMR serves as the “source of truth” for patient information, ensuring that the data in the imaging system aligns with the most up-to-date records. AI acts as a vigilant detective, flagging any discrepancies that could lead to errors during the migration process.
Cleansing and Standardizing Information
One of the biggest challenges in DICOM data migration is dealing with inconsistent or incomplete data. Over time, PACS databases can accumulate a significant amount of “dirty” data, which can compromise the accuracy and reliability of the new system. AI can play a crucial role in cleansing and standardizing this data. By using natural language processing (NLP) and computer vision, AI algorithms can analyze study and series descriptions, ensuring that they are correct, complete, and consistent. This involves filling in missing fields, correcting errors, and standardizing terminology to ensure that all the data is uniform and easily searchable.
Imagine a scenario where a patient is initially admitted to the hospital under temporary credentials due to a trauma. Later, their identity is confirmed, and the EMR is updated. Without AI, the original imaging files might retain the outdated information. AI can automatically reconcile these discrepancies, ensuring that the correct patient information is associated with the corresponding images.
Building a Solid Foundation
The goal of DICOM data migration is not just to move data from one system to another, but to improve the quality and usability of that data. AI can help achieve this goal by optimizing the way the data is organized and structured in the new system.
By using machine learning, AI algorithms can classify and categorize images based on their content and clinical relevance. This allows for more efficient searching and retrieval of images, which can improve diagnostic accuracy and reduce the time it takes for radiologists to review studies.
The integration of AI into DICOM data migration is more than just a technological upgrade; it represents a fundamental shift in how healthcare organizations manage their data. By automating and streamlining the migration process, AI frees up valuable IT resources, reduces the risk of errors, and improves the quality of the data. As AI continues to evolve, its role in medical imaging will only become more prominent, paving the way for a more efficient, accurate, and patient-centered healthcare system.
This concludes part one of our exploration into AI-enabled DICOM data migration, showcasing how Laitek and Enlitic’s collaboration is transforming initial data assessment and planning. From AI-powered data discovery to intelligent data mapping, these advancements are setting a new standard for efficiency and accuracy in large-scale data projects. To read the full article and learn more, visit www.enlitic.com/migration. Join us there for the next article in this series, where we’ll delve into how AI is revolutionizing the migration process itself, tackling the complex task of moving and converting vast amounts of DICOM data with unprecedented speed and accuracy.